Reducing Surgical Wait Times: A Collaborative Approach with Community Hospitals
Presenter: Suting Yang, Senior Methodologist, The Hospital for Sick Children
Abstract: The prolonged wait time for elective surgeries has significantly worsened since the start of the pandemic – as of August 2023, over 50% of the elective surgery wait times were exceeding the expected timeframe, and more than 10% of patients have been on the waitlist for over two years. To address this, The Hospital for Sick Children (SickKids) aims to work alongside community hospital networks to establish local sub-hubs for providing specific surgical services. The goal is to identify the most suitable hospital(s) for each service to partner with, ensuring timely and equitable access to surgical care for all patients. Services for this initiative include orthopedics, dentistry, ophthalmology, plastic surgery, otolaryngology, general surgery, and urology. Our spatial analysis consists of two parts: (1) a social determinants analysis via Generalized Estimating Equation (GEE) Logistic Regression to explore how social factors relate to wait times in Greater Toronto Area (GTA), and (2) a multi-criteria decision analysis to evaluate hospitals and derive their utility scores. The findings indicate that historical surgeries show no significant association between social determinants and wait times in completed surgeries, though surgery-to-waitlist ratio suggests an under-representation for highly marginalized groups. Additionally, our analysis identifies a community hospital that consistently ranks highly across services. We recommend connecting with this hospital to ensure adequate healthcare human resources for cooperation, which in turn would help in mitigating the surgical backlog and reducing wait times.
|
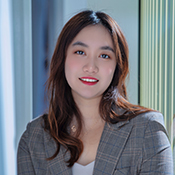 |
Suting Yang is a healthcare engineering professional with hands-on experiences in big data analytics, forecasting, simulation, and optimization in healthcare. She holds a Master of Applied Science in Industrial Engineering, specializing in healthcare analytics from the University of Toronto. Throughout her career, Suting has worked with multiple healthcare agents and hospitals, collaborating on data analytics projects. Suting is currently a Senior Methodologist at The Hospital for Sick Children, providing advanced analytics support to different parts of the organization.
|
|
|
Nurse Workload Balancing Using Real-Time Location Data
Presenter: Vedat Verter, Smith School of Business, Queen’s University, Canada
Abstract: Sustained inequities in the workload distribution can lead to increased stress and reduced job satisfaction, high turnover and shortages in the nursing team. These imply that healthcare quality could also eventually suffer from the imbalances in nursing workload. We develop a data-driven analytical framework to achieve balanced nurse workloads by optimizing the nurse-patient assignment decisions at the beginning of every shift. To this end, we utilize an extensive data set collected by a real-time location system installed in the surgical services department of a large tertiary hospital. This enabled us to track the care providers as well as the surgical patients through their journey from the emergency department to the operating room, and the surgical ward. The nurse workload is modeled as a multi-attribute, multilinear function, where the significance of each attribute (for the nurse manager) is elicited using an inverse optimization procedure integrated into a clustering method. This involves inverse optimization with a nonlinear integer original problem, which has not been well studied in the literature. The nurse workload balancing problem is then formulated for the upcoming shift, whereby the nurse-patient assignment decisions constitute the primary lever. This requires deploying the proposed dynamic panel-data model to predict each patient’s required direct care. We also robustify the model to incorporate the uncertainties in the attribute weights. Through a real-life case-study we show that the proposed approach would have reduced the maximum travel distance by 50% - 77%. In addition, the average ranges for total direct care, maximum travel distance, and number of assigned patients decrease by 65%, 45%, and 31%, respectively.
|
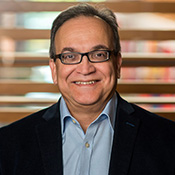 |
Vedat Verter is a Professor and Stephen J.R. Smith Chair of Management Analytics at Queen’s University. He specializes on the application of operations research and data analytics for assisting policy makers in the public sector. His primary areas of research are socially responsible supply chains and healthcare analytics. In the area of healthcare, he focuses on preventive, primary, emergency, acute and chronic care processes, as well as their interaction. His earlier work focused on service chain design and hazardous materials logistics. Professor Verter’s work in these four areas culminated to 80+ research articles in refereed journals and 20 book chapters. His research is well recognized through invited presentations around the globe.
|
|
|
Decoding Patient Trust: Navigating Precision and Peer Acceptance in AI Gatekeeper Healthcare Systems
Presenter: Susan Lu, Purdue University
Abstract: This study investigates the dynamics between humans and algorithms in healthcare, focusing on patient-AI gatekeeper interactions where the AI recommends doctors based on patient-provided symptoms. Through a five-month field experiment in a healthcare organization, patients were randomly assigned to four groups, each receiving varied AI recommendation scenarios: (1) recommendation only; (2) recommendation with prediction accuracy; (3) recommendation with peer acceptance; and (4) recommendation with both prediction accuracy and peer acceptance. Our findings indicate a general lack of trust in AI recommendations among patients. Despite this, a positive correlation emerges between patient acceptance and the separate and combined influences of information on prediction accuracy and peer acceptance. Patients willingly embrace recommendations with high peer acceptance, even with lower accuracy. Those actively engaged in providing detailed symptom information are less affected by algorithmic accuracy and peer decisions, while new patients are more receptive to algorithmic influences than existing ones. In a broader context, human inclination leans towards conformity to peer choices over reliance on algorithmic precision. Patients lacking prior healthcare process knowledge or struggling to articulate their health conditions tend to defer to others' choices and algorithmic accuracy. This study unveils nuanced dynamics shaping trust and reliance on AI in healthcare, offering insights into the evolving landscape of human-AI interactions.
|
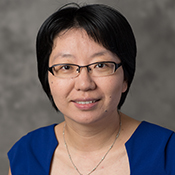 |
Dr. Susan Lu is the Gerald Lyles Rising Star Professor of Management at Purdue University. Her research centers on healthcare operations, with an emphasis on nursing home operations and cardiac care delivery. She provided expert testimony in the US Senate and serves as associate editors for top operations management journals.
|
|
|
Physician Rostering with Downstream Capacity Constraints
Presenter: Arik Senderovich, York University; University of Toronto, Rotman School of Management
Abstract: We study a physician rostering problem that commonly arises in outpatient hospitals whereby physicians are scheduled to weekly sessions during which they examine and consult patients, which is a NP-hard problem. Rostering the sessions requires coordinating not only the resources necessary for conducting the examinations, but also considering the following (downstream) treatment activities which are both resource- and time-intensive, and where delays occur. We formulate a Mixed Integer Linear Programming to solve the rostering problem and develop a predictive model to estimate the infusion load. We collaborate with a large hospital that specializes in cancer treatment and develop an interactive interface that optimizes the physicians' roster (i.e., sessions) to improve patient flow and the utilization of resources. We conduct numerical experiments using historical data to assess the accuracy of the infusion load predictions and the potential improvement in different service quality metrics that can be obtained by optimizing the roster.
|
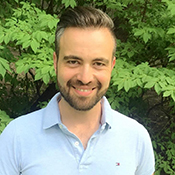
|
Arik Senderovich is an Assistant Professor at the School of Information Technologies (ITEC) at York University and a Status-Only Assistant Professor at Rotman School of Management (University of Toronto). His research intersects Operations Management, Data Science, and Artificial Intelligence, focusing on developing methodologies for modeling complex, congested environments like hospitals and public transportation systems.
Senderovich holds a PhD in Data Science, an MSc in Statistics, and a BSc in Industrial Engineering and Management, all from the Technion. He has been recognized with awards for his dissertation and paper at the International Conference on Business Process Management (BPM). He is also the co-founder of a U of T based start-up company, SiMLQ, that provides simulation- and ML-driven solutions to business analytics in congested queueing systems.
|
|
|
Resilience and Adaptation: Assessing the Effects of COVID-19 on Hospital Efficiency and Quality
Presenter: Adam Diamant, Associate Professor of Operations Management and Information Systems, Schulich School of Business
Abstract: This study examines the impact of COVID-19 on different dimensions of hospital operations, which we categorize into three main areas based on the extant literature: clinical efficiency, technical proficiency, and experiential quality. Utilizing a dataset of over 250,000 patient admissions from 15 hospitals in Ontario, Canada between January 1, 2019 and August 31, 2021, we first use data envelopment analysis (DEA) to assess hospital performance based on input variables such as readmission rate, mortality rate, length-of-hospital stay, the number of ED visits and surgeries, and wait time metrics for various inpatient services (e.g., radiography, echocardiograms). We then use the estimated DEA values as dependent variables in a fixed-effect, difference-in-differences (DiD) regression model with heterogeneous treatment times to explore the causal relationship between the onset of COVID-19 and a hospital's operational effectiveness in these three different dimensions. Our findings reveal a significant negative impact on clinical efficiency which can be attributed to the COVID-19 pandemic, whereas technical proficiency and patient experience exhibit resilience amidst well-documented operational challenges. The consistency of these results across various robustness checks and alternative empirical specifications underscores their reliability. Consequently, this study deepens our understanding of the implications that COVID-19 had on hospital operations and provides valuable insights for healthcare management strategies in the face of such disruptions.
|
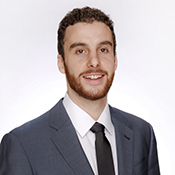 |
Adam Diamant is an Associate Professor of Operations Management and Information Systems at the Schulich School of Business and is a York University Research Chair in Managing AI-Driven Technologies in Health Care. His research uses mathematical techniques and data-driven methodologies to study and model complex, large-scale systems in health care to obtain insights for better administrative and operational decision making.
|
|
|
The Impact of Information-Granularity and Prioritization on Patients’ Care Modality Choice
Presenters: Ricky Roet-Green, Associate Professor of Operations Management, Simon Business School, University of Rochester
Abstract: The past few years have witnessed a significant expansion in telemedicine adoption by healthcare providers. On one hand, telemedicine has the potential to increase the accessibility of medical appointments. On the other hand, due to the limitation of remote diagnostic and treatment methods, telemedicine may be insufficient for patients' treatment needs and necessitate subsequent in-person follow-up visits. To better understand this trade-off, we model the healthcare system as a queueing network providing two types of service: telemedicine and in-person consultations. We assume that an in-person visit guarantees successful treatment, whereas a telemedicine visit may fail to meet the treatment needs with a probability that is contingent on individual patient characteristics. We formulate patients' strategic choices between these care modalities as a queueing game and characterize the game-theoretic equilibrium and the socially optimal patients' choices. We further examine how improving patients' understanding of their telemedicine suitability through predictive analytics at the online triage stage affects system performance. We find that increasing information granularity maximizes the stability region of the system but may not always be optimal in reducing the average waiting time. This limitation, however, can be overcome by simultaneously deploying a priority rule that induces the social optimum under specific conditions. Finally, leveraging real-world data from a large academic hospital in the United States, we perform a comprehensive case study that encompasses both the development of a prediction model for in-person follow-up needs and the implementation of effective information provision and patient scheduling strategies.
|
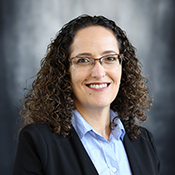
|
Ricky Roet-Green is an Associate Professor of Operations Management at Simon Business School, University of Rochester. Her research interests lie in modeling and analyzing the behavior of strategic customers in congestion-prone environments, particularly in service systems. Her work integrates queueing theory, game theory, and mechanism design, aiming to understand customers’ decisions and optimize system performance in terms of revenue and social welfare maximization.
|
|
Lin Zang is a 4th year PhD student from Simon Business School, University of Rochester, under the supervision of Prof. Ricky Roet-Green. Her research interests lie in analyzing the impact of various types of information on customers' strategic joining decisions to different queueing systems. Her work combines techniques from queueing theory, game theory, and mechanism design to improve the performance of queuing systems and customers’ welfare.rof. Ricky Roet-Green. Her research interests lie in analyzing the impact of various types of information on customers' strategic joining decisions to different queueing systems. Her work combines techniques from queueing theory, game theory, and mechanism design to improve the performance of queuing systems and customers’ welfare.
|
|
|
Interpretable Models for Predicting Heart Attack Incidence Using Demographic Data
Presenter: Amir Rastpour, Faculty of Business and Information Technology, University of Ontario Institute of Technology
Abstract: To be effective, heart attack treatment procedures must start as soon as possible after the onset of symptoms. Therefore, heart attack treatment centers should be strategically located to maximize timely accessibility for potential patients. To achieve this goal, health care decision makers need to have accurate predictions of the number of heart attack patients in high spatial resolution. We develop interpretable, as opposed to black box, models for predicting the number of heart attack patients in granular geographical areas. Our models are interpretable as they satisfy some constraints originating from the medical literature and common sense: older people have a higher chance of heart attack, predictions are non-negative, more populous geographical areas have a higher expected number of patients, and the per-person rate of heart attack must remain fixed if we change our spatial unit. Our predictions are granular enough so that decision makers can use them to obtain accurate estimates of demand within any desired travel time radius from healthcare providers. The proposed models are extensions of the Poisson and linear regression models. We use 10 years' worth of empirical heart attack data from Alberta, Canada, and show that our interpretable models outperform the black box models.
|
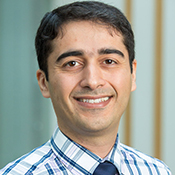
|
Amir Rastpour is an Associate Professor of Operations Management at Ontario Tech University. He is interested in improving the healthcare system using queuing theory, statistical and machine learning methods. He has dealt with the healthcare system both at the planning level (such as demand prediction for heart attack treatment facilities and finding promising locations for these facilities) and at the operational level (such as emergency medical services operations).
|
|
|
Uncovering Hidden Adverse Side Effects of GLP-1 Receptor Agonists Used for Obesity Treatment Through an Integrated Social Media Analytics Research
Presenter: Prof. Abraham (Avi) Seidmann, Questrom School Of Business, Boston University
Abstract: GLP-1 receptor agonists are presently widely prescribed for treating obesity in growing numbers in the US and abroad. Although clinical trials are designed to capture critical ASEs, many other ASEs are only discovered after marketing and might pose a severe health threat. This is potentially due to unexpected drug interactions, idiosyncratic lifestyles, or some deviations from the recommended intake regime. Our research aims to develop a digital methodology that applies AI-driven computational techniques to uncover hidden ASEs of GLP-1 receptor agonists based on social media data, published research papers, reports by drug manufacturers, and the rapidly growing ChatGPT knowledge. Our study showed that manufacturers did not report 21 adverse side effects (ASEs) associated with GLP-1 receptor agonists mentioned on social media or in clinical trials. We constructed a network of interconnected ASEs that revealed clinically relevant clusters of co-occurring ASEs. Our Graph Convolutional Model was also trained to predict ASE frequency with high accuracy, achieving an average F1 Score of 0.79 and an AUC of 0.82. Additionally, we conducted sentiment analysis to gain insights into crucial public perceptions and usage concerns regarding these medications. Our research underscores the significance of utilizing advanced AI techniques to uncover valuable insights from social media data in pharmacovigilance efforts. By implementing our "early warning" methodology, regulators can identify hidden adverse side effects early, improving public safety and informing treatment decisions more promptly.
|
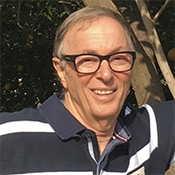 |
Abraham (Avi) Seidmann is the Everett W. Lord Distinguished Faculty Scholar of Information Systems and an Associate Research Director for Health Analytics and Digital Health at the Questrom Digital Business Institute. He is a national expert in Business and Medical Management, social media and digital Health, and Telemedicine. Prof Seidmann has led clinical and economic research in these areas for over 25 years. He has published over one hundred research articles and has over 9,900 research citations. In October 2012, he was named a “Distinguished Fellow” by the Information Systems Society of the Institute of Operations Research and Management Sciences (INFORMS).
|
|
|
Making AI Impactful in Healthcare
Presenter: Dr. Soroush Saghafian, Associate Professor, Harvard University
Abstract: There is an increasing amount of evidence that Machine Learning and Artificial Intelligent algorithms can be used to enhance clinical care. In this talk, I address two critical aspects that can significantly improve the impact of such algorithms in healthcare practices: (1) moving beyond associations, and creating algorithms capable of causal reasoning under ambiguity, and (2) a human-algorithm “centaur” model of care and decision-making, in which the power of human intuition is combined with the outstanding capabilities of algorithms. I describe our latest research at the Public Impact Analytics Science Lab (PIAS-Lab) at Harvard on these subjects, and discuss findings based on our various collaborations with the Mayo Clinic as well as some other public and private organizations.
|
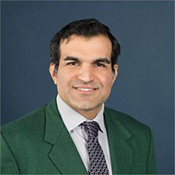
|
Dr. Soroush Saghafian is an Associate Professor at Harvard University. He is the founder and director of the Public Impact Analytics Science Lab (PIAS-Lab) at Harvard, which is devoted to advancing and applying the science of analytics for solving societal problems that can have public impact. He also serves as a core faculty member for the Harvard Center for Health Decision Science, a faculty affiliate for (a) the Harvard Data Science Initiative, (b) Harvard Ph.D. Program in Health Policy, (c) the Harvard Mossavar-Rahmani Center for Business and Government, (d) the Harvard Belfer Center for Science and Technology, and (e) the Harvard Center for Public Leadership. He is also an associate faculty member at the Harvard Ariadne Labs (a pioneer lab in health systems innovation) and holds a research collaborator position at the Massachusetts General Hospital.
|
|
|
Work Design and Scheduling for Dialysis Clinics
Presenter:Vince Slaugh, Cornell University
Abstract: Over 500,000 people in North America rely on hemodialysis as a life-critical medical treatment for advanced kidney failure. We study a dialysis clinic's daily planning and operation, focusing on the joint problem of scheduling patients and designing the work structure for patient care technicians (PCTs) who typically serve one “pod” of four chairs. Their main tasks are “putting on” and “taking off” patients before and after treatments of predetermined lengths. The dialysis clinic seeks to minimize two correlated objectives: the completion time for the last patient and the ratio of labor hours to patients served. We provide analytical results for three interpretable schemes that define patient scheduling and how PCTs interact with patients: push, in which PCTs put on patients as soon as chairs are available; interlaced, in which PCTs delay put-ons early in the shift to allow space for future put-ons to happen immediately after take-offs on each chair; and tandem, in which two PCTs specialize in put-ons or take-offs for a combined pod of eight chairs. In particular, we show that the interlaced scheme is optimal among solo schemes. Also, although the tandem scheme may have longer last-patient completion times than the interlaced scheme, it maximizes labor efficiency. These insights hold numerically with realistic distributions of heterogeneous patient treatment times. Our analytical and numerical insights have inspired changes currently being implemented in a test market for a large dialysis clinic network.
|
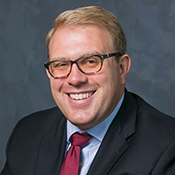
|
Vince Slaugh is an assistant professor of service operations management at the Nolan School of Hotel Administration in the SC Johnson College of Business at Cornell University. He uses stochastic models to study strategic service workforce decisions and provide tactical decision support for frontline service work. Care-oriented operations, including hotel housekeeping, nursing homes, and the child welfare system, motivate his research.
|
|
|
Resource-Neutral Approaches to Improving Operating Room Efficiency: Lessons Learned from a Quaternary Hospital Network
Presenter: Johanna Pinto Lee, Resident surgeon, Department of Surgery, University of Toronto; MBA Candidate, Rotman School of Management
Abstract: In Canada’s single-payer healthcare system available resources are finite; hospital institutions are consistently asked to do more with less. Operating room (OR) inefficiencies are an excellent target for optimization, especially after the COVID-19 pandemic created a substantial surgical backlog. These pressures presented an opportunity to increase OR efficiency to support backlog clearance and increase surgical access for patients waiting for their surgical care. We performed a quality-improvement initiative to redesign OR services at the University Health Network (UHN), the largest hospital network in Canada. Lessons learned from the initiative demonstrate how efficient, resource-neutral changes create the groundwork for improving OR efficiency. By learning from COVID-19, a period with more than normal strain on resources, UHN was able to increase OR use (using existing resources) and accurately model for future demands. The UHN experience shows that by first analyzing current OR use, OR efficiency can be optimizing by: 1. Separating OR scheduled and emergent activity; 2. Coordinating OR utilization to inpatient bed availability; 3. Designing better tools to accurately capture OR wait lists in alignment to the surgical backlog due to the COVID-19 pandemic; and 4. Restructuring OR scheduling and governance process. Resource neutral changes at UHN had an outsized impact: UHN ORs are running at 122% capacity compared to 2019-2020, completing 16% more procedures on average. Lessons learned from the UHN experience can be extrapolated to other hospital networks to facilitate a multipronged approach to clearing the surgical backlog post-COVID.
|
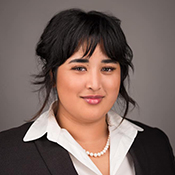
|
Johanna Pinto Lee is a resident surgeon in the Division of General Surgery at the University of Toronto and an MBA candidate at the Rotman School of Management. Prior to her surgical training, Johanna served as a policy analyst to the Chief Public Health Officer of Canada, Dr. Theresa Tam. Johanna is fascinated by complex systems, whether in business or healthcare, and is passionate about developing systems-level strategies for effective, equitable healthcare.
|
|
|
Helping the Captive Audience: Advance Notice of Diagnostic Service for Hospital Inpatients
Presenter: Nan Liu, Department of Business Analytics, Carroll School of Management, Boston College
Abstract: How to schedule patients is a classic operations management problem in healthcare. There are two standard paradigms of dynamic scheduling: advance scheduling and allocation scheduling. Advance scheduling provides patients with specific service times, letting patients enjoy the most convenience, whereas allocation scheduling puts patients on a wait list and gives providers the flexibility of calling patients for service at the last minute. Hospital inpatient diagnostic service is usually managed via allocation scheduling: inpatients are treated as the “captive audience” on-demand, and they are notified only when hospital diagnostic service capacity is available. This arrangement causes significant chaos and inefficiencies in operations. We propose an innovative scheduling policy, called advance notice, to manage hospital diagnostic service. Patients are placed in a common queue waiting to be called for service, and they will be provided both a fixed preparation time and a guaranteed service time window in advance (neither a last-minute notice nor an exact service time in the future). The advance notice policy represents a new scheduling paradigm to strike a fine balance between the two classic ones: it enjoys the benefit of allocation scheduling (giving the provider flexibility in using her capacity) and that of advance scheduling (reducing patient online waiting). We formulate and analyze a Markov Decision Process model to study advance notice decisions. Our numerical study, populated by data from a large academic medical center in the U.S., demonstrates significant improvement in operational efficiency by switching from current practice to adopting our advance notice policy.
|
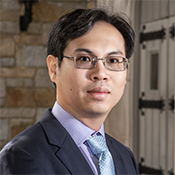
|
Nan Liu is an Associate Professor of Business Analytics and William S. McKiernan Family Faculty Fellow at Carroll School of Management, Boston College. His research interests span service operations management, consumer behavior, and health policy. His recent work focuses on innovating strategies to match health service supply with demand, with the goal of improving access, efficiency, equity, and quality.
|
|
|
Reducing Diagnostic Imaging Wait Times: Lessons from a Large-Scale Empirical Analysis
Presenter: Benjamin Ravenscroft, University of Waterloo, Department of Management Sciences
Abstract: Wait times for diagnostic imaging, such as MRIs, have become an increasingly pressing issue due to demand outpacing supply in many countries. Not only do long wait times negatively affect patient satisfaction, but they also have detrimental effects on prognosis and can have serious downstream economic consequences. Specifically in Canada, wait times for semi-urgent and non-urgent patients can be 2-4 times the Canadian Association of Radiologists benchmarks. Considering that service duration is a key factor affecting system throughput, we focus on investigating the determinants of procedures’ service times and examine how these factors might be leveraged to increase throughput and decrease wait times. Using large-scale patient-level MRI services data from 66 hospitals in Ontario, we show that several covariates, including sequencing of similar procedures, the shift during which a procedure is performed, and the service times of the preceding patients' procedures, significantly affect MRI service duration. For example, we show that sequencing similar service types reduces subsequent procedures’ durations. In particular, we observe that if a procedure is scheduled to occur after another procedure of the same body type, its duration will be roughly 4.2% shorter than the previous procedure. Furthermore, we conduct counterfactual analysis using a heuristic scheduling algorithm that aims to maximize the beneficial effects of sequencing in combination with trace-driven simulation. These simulations demonstrate that by considering sequencing when scheduling semi- and non-urgent MRI examinations, the daily number of procedures performed can be increased by up to 22% in some hospitals, without increasing total worked hours.
|
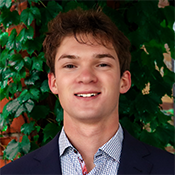
|
Benjamin Ravenscroft is a MASc student in the Department of Management Sciences at the University of Waterloo, with a BSc in Computer Science from Queen’s University. His research interests include data-driven modeling and empirical analysis of service systems with a focus on the healthcare sector.
|
|
|